Understanding Clip Skip Stable Diffusion
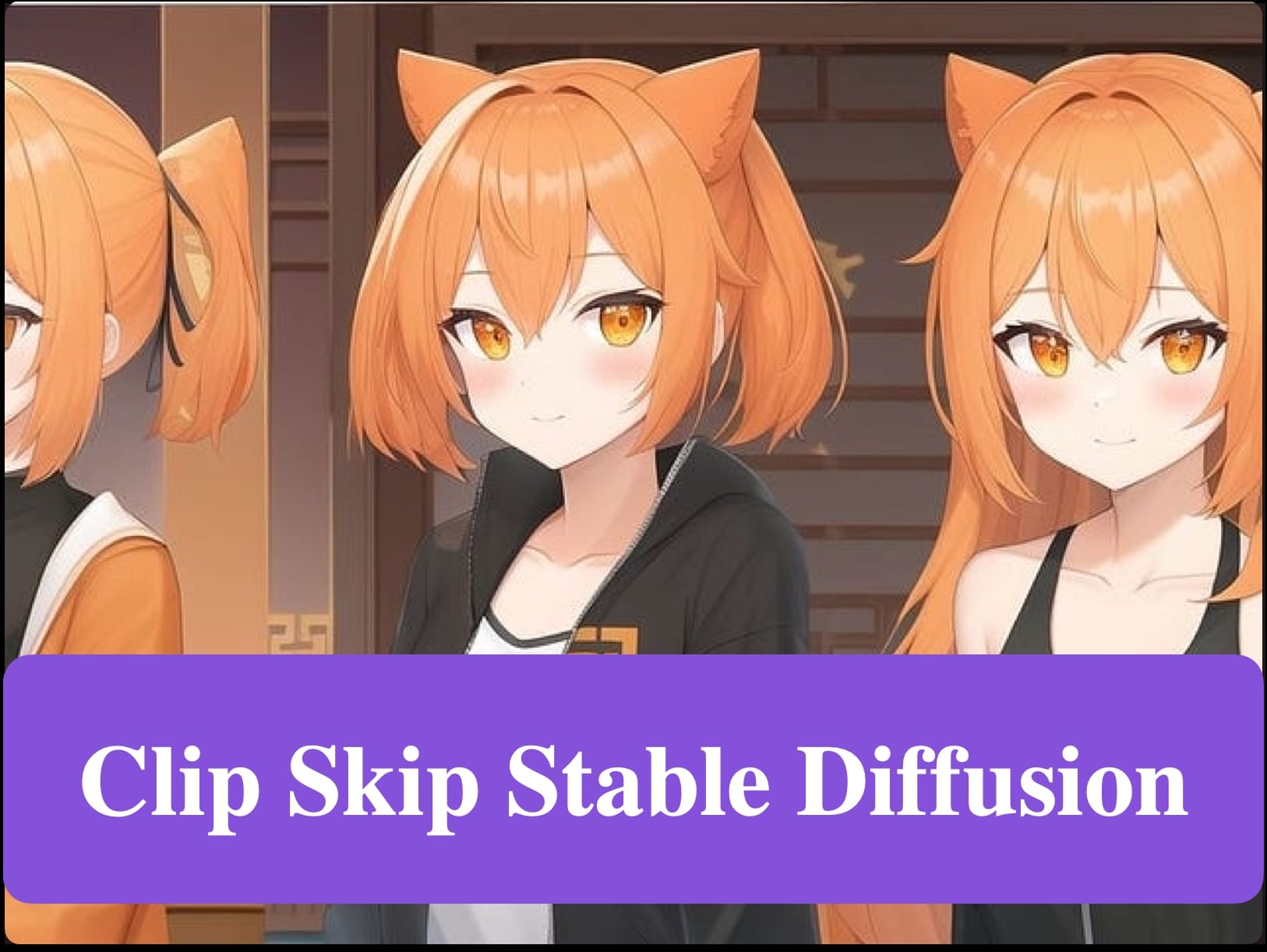
Learn about clip skip stable diffusion and its significance in the industry. Explore our blog for in-depth information.
Clip Skip Stable Diffusion is a cutting-edge technique that has revolutionized the field of image generation. By combining the power of Clip Skip and stable diffusion, this approach allows for the creation of highly realistic and diverse images. In this blog, we will delve into the concept of Clip Skip, explore its role in stable diffusion models, and discuss the importance of understanding this technique while working with stable diffusion. We will also examine different stable diffusion models, evaluate their effectiveness, and explore the process of merging models for enhanced performance. Additionally, we will decode stable diffusion model file formats and diversify with other model types. So, let’s dive into the fascinating world of Clip Skip Stable Diffusion.
Exploring Clip Skip in Stable Diffusion
Clip Skip is a technique that plays a crucial role in stable diffusion algorithms, particularly in the image generation process. It allows for the skipping of certain pixels or blocks during the compression process, resulting in faster and more efficient compression. By reducing the amount of data that needs to be processed, Clip Skip contributes to improved compression performance. In the context of stable diffusion, Clip Skip helps in minimizing artifacts and enhancing the overall quality of the generated images.
The Concept of Clip Skip
Clip Skip operates in a special way, interacting with different layers in the stable diffusion model. At the last layer of the text encoder, Clip Skip influences the image generation process. It facilitates the generation of images based on the corresponding text prompt, resulting in a more customized output. By skipping certain sub tags or categories, Clip Skip adds a layer of specialization to the image generation process. This functionality allows for the creation of diverse and targeted images, catering to specific requirements and use cases.
The Role of Clip Skip in Stable Diffusion
Clip Skip plays a significant role in stable diffusion models. It is a technique used to improve the performance of image and video compression in stable diffusion algorithms. By allowing the skipping of certain pixels or blocks, Clip Skip reduces the amount of data that needs to be processed, resulting in faster and more efficient compression. This technique also helps to reduce artifacts and enhance the overall quality of compressed images and videos. Understanding how Clip Skip works is essential for developers to optimize their compression algorithms and improve the user experience for their customers.


Delving into Stable Diffusion Models
Stable diffusion models are a key component of the stable diffusion process in AI image generation. These models form the foundation for generating realistic and diverse images. In this section, we will explore the basics of stable diffusion models, popular stable diffusion models, and the process of creating these models. Understanding the different types of stable diffusion models and their characteristics is crucial for achieving optimal results in image generation.
The Basics of Stable Diffusion Models
Distilled versions of the Stable Diffusion (SD) model, represent efforts to create more efficient, often smaller versions of the original SD model. These distilled models aim to retain as much of the original model’s capabilities as possible while being faster or more resource-efficient. The creation of these distilled models is a response to the growing need for more accessible and efficient AI models. They allow broader usage across various platforms and devices, especially where computational resources are a limiting factor.
Stable diffusion models, such as the clip model, are essential in NLP. These models are used to represent long-range dependencies and capture sequential information effectively. The clip stage, separated into clip layers, enables the model to learn hierarchical features, making it useful for various applications in the internet, including anime embedding and sd. Understanding stable diffusion models is crucial for anyone working with NLP and wanting to leverage the power of clipskip for their projects.
Popular Stable Diffusion Models/’
Stable diffusion models, like the clip model, are essential in NLP for understanding information propagation. These models incorporate clip layers to represent how content spreads in a network. The young man is an example of an individual at the beginning of the clip stage, where the diffusion process starts. Understanding these models is crucial for analyzing information flow on the internet and in applications such as anime embedding and sd.
How Stable Diffusion Models are Created
Stable diffusion models are created using various layers of the clip model. These layers encapsulate the clip stage’s potential and its impact on diffusion. By incorporating young man’s SD in the process, these models can accurately represent the internet’s embedding of clipskip. The blog delves into how these models effectively capture the essence of anime clips, contributing to a deeper understanding of clip skip stable diffusion processes.


Evaluating the Effectiveness of Different Stable Diffusion Models
When evaluating stable diffusion models, it’s crucial to consider a variety of factors. The clip model and its effectiveness should be analyzed thoroughly. Additionally, understanding the impact of clip layers and embedding in the diffusion process is essential. Evaluating the role of sd and its influence on diffusion is also significant. Furthermore, exploring the internet and its relevance to stable diffusion models can provide valuable insights. Assessing the different stages of clip and their contributions to the overall model effectiveness is a critical aspect as well.
Comparing Stable Diffusion v1.4 and v1.5
Stable Diffusion v1.5 introduces improvements in clip model and clip layers compared to v1.4. The young man’s anime embedding is more efficient in v1.5, enhancing sd on the internet. These changes make v1.5 a significant upgrade from v1.4, offering better performance and capabilities for users.
The Impact of Realistic Vision and DreamShaper
Realistic vision influences the development of DreamShaper. As a result, young man can perceive and analyze scenes in an immersive manner. The clip model integrates clip layers and clip stage, creating a seamless experience. This impacts not only anime creation but also the embedding of sd content on the internet. The blog explores how realistic vision affects the creation and sharing of clipskip content, shaping the future of digital storytelling.
Analyzing the SDXL Model and Anything V3
The SDXL model processes text, image, and audio inputs and incorporates Clip Skip to enhance results. Similarly, the Anything V3 model, a variant of the Omega model, is optimized for text generation and utilizes Clip Skip for improved performance. The SDXL model is involved in image generation, stable diffusion, and text prompts, while the Anything V3 model follows a different process, generating unique outputs. Both models demonstrate the effectiveness of Clip Skip in their respective tasks.


Merging Two Models for Enhanced Performance
To enhance performance, merging two models can be highly beneficial. By combining the clip model with the young man, clip stage, and clip layers, enhanced results can be achieved. This fusion leverages the strengths of both models, leading to better outcomes in various applications such as anime, embedding, and internet content. Additionally, the implementation of sd and clipskip further refines the merged model, ensuring improved stability and diffusion.
The Process of Merging Models
Integrating the text model with the image model creates a unified AI model with enhanced capabilities. The resulting model undergoes stable diffusion, ensuring seamless integration of the base models’ features. This merging process requires careful consideration of the base model’s attributes and compatibility to ensure optimal performance. Additionally, it enriches the image generation process by giving access to a broader dataset. The merged model’s text encoder and image generation process work collaboratively, resulting in specialized outputs.
Example of a Successful Model Merge
By integrating various base models, a successful model merge produces diverse and high-quality image generation. The merging process benefits from the diffusion characteristics of the integrated base models, resulting in efficient image generation. Users can achieve specialized image output catering to specific requirements and use cases by merging two models. The resulting model demonstrates enhanced performance, providing unique and tailored image outputs. Successful model merges leverage stable diffusion, resulting in improved and efficient image generation.

Decoding Stable Diffusions Model File Formats
Understanding the file formats in stable diffusion models is crucial for effective analysis. The clip model utilizes specific formats for data storage representing various features at each clip stage. These formats incorporate clip layers and embedding mechanisms, providing comprehensive insights into the underlying data. Differentiating between sd, internet, and clipskip formats is essential for interpreting the information accurately.
Difference between Pruned, Full and EMA-only Models
Pruned models simplify by removing unnecessary network parts, while full models retain all original components. EMA-only models use exponential moving averages to smooth training and reduce data noise. Clip Skip Stable Diffusion (CSSD) combines pruned and full models, utilizing EMA for improved performance. CSSD offers better efficiency than traditional models, making it promising for machine learning research. This method enhances computational performance, and its applications span beyond traditional methods.
Understanding Fp16 and fp32 Models and Safetensor Models
Fp16 and fp32 models feature varying precision levels, impacting stable diffusion model performance and resource utilization. Safetensor models prioritize safety, enhancing reliability and stability. Fp16 models optimize memory usage and computational efficiency using half-precision floating-point format, while fp32 models offer higher precision and accuracy with single-precision floating-point format. Understanding these model characteristics is crucial for informed deployment decisions, ensuring efficient and reliable stable diffusion model operations.

Diversifying with Other Model Types
Exploring diverse model types can enhance and broaden the results obtained. It provides a wider perspective and understanding of image generation, opening up new possibilities in the process. Different model types offer unique viewpoints and experimenting with them can lead to unexpected and insightful outcomes. This diversification can lead to improved and specialized results in the image generation process.
How essential is it to understand Clip Skip while working with Stable Diffusion?
Understanding the concept of Clip Skip is crucial when it comes to achieving stable diffusion results. It plays a significant role in generating successful stable diffusion outcomes and navigating the process effectively. Mastering the use of Clip Skip is essential to ensure that stable diffusion results are achieved.
Conclusion
In conclusion, understanding Clip Skip Stable Diffusion is crucial for anyone working with stable diffusion models. Clip Skip plays a significant role in enhancing the functionality and effectiveness of these models. It allows for better results and performance by skipping irrelevant information during diffusion. Additionally, merging two models can further enhance the performance of stable diffusion models, as demonstrated by successful examples. It’s also essential to familiarize oneself with different model file formats, such as pruned, full, EMA-only models, and understand the differences between fp16, fp32 models, and Safetensor models. Lastly, while working with stable diffusion, having a comprehensive understanding of Clip Skip is vital for achieving optimal outcomes. So dive into stable diffusion, explore Clip Skip, and unlock the full potential of these models for your projects.
novita.ai provides Stable Diffusion API and hundreds of fast and cheapest AI image generation APIs for 10,000 models.🎯 Fastest generation in just 2s, Pay-As-You-Go, a minimum of $0.0015 for each standard image, you can add your own models and avoid GPU maintenance. Free to share open-source extensions.
Recommended reading